Once the various AI models became more advanced and capable of handling complex tasks, it was a good sign for marketers that one big task could be handed over to AI: customer segmentation and data analysis. But the more we go, the more professionals realize that too much AI doesn’t necessarily mean that their work is more efficient. Now, talking about customer segmentation, AI models can really excel in this domain: feed them with a list of data and they will analyze it. However, let’s put a question mark here, as not all of us believe that segmentation based on numbers is a good enough solution for understanding the customers at the level of personalized marketing.
Authentic Data about Real Audiences
Proxy servers have become an invaluable technical tool to access the real internet experience of target customers. They enable marketers to bypass geographic restrictions and content filters that would otherwise impede research. For example, if certain social sites or e-commerce content are region-locked, a proxy can route your connection through a local IP, letting you view the website as if you were in that country.
This means a team in Germany can study an American customer’s online journey (or vice-versa) without false barriers. Similarly, proxies help avoid bot-blockers; Many websites deploy anti-scraping measures like CAPTCHAs or IP bans against automated crawlers. Using rotating or high-anonymity proxies helps companies collect data at scale without getting cut off, by making their traffic appear like ordinary users’ traffic. Moreover, there are so many offers from reliable platforms that anyone could get a free proxy and make their research several times more effective with a snap of their fingers.
Importantly, proxies (especially residential proxies) make your data collection look authentic. Residential proxy networks route requests through real user devices/IPs, so that your browsing appears to come from a normal consumer in the target demographic. This allows businesses to gather raw behavioral data that reflects what real customers see and do online, rather than a sanitized or blocked view. By combining data from multiple regions and user contexts (all accessed via proxies), marketers can build segmentation models on a foundation of rich, unbiased data. The end result is smarter segmentation: decisions based on how actual customers behave across geographies and channels, not just the easily reachable subset of users.
Why Understanding Psychographics is Critical
Effective segmentation isn’t just about who the customer is in a demographic sense but why they behave the way they do. This is where psychographics come into play. Psychographic segmentation focuses on people’s values, attitudes, lifestyles, and personalities rather than surface-level traits. In other words, it groups people by shared motivations and mindsets, not just by age or income.
Marketers have long sorted audiences by demographics, which does provide useful context. But relying only on demographics assumes everyone in a given category thinks and acts alike – an obvious oversimplification, right?. Two 30-year-old women may have vastly different priorities if one is a budget-conscious minimalist and the other is a status-driven trendsetter. Traditional segments wouldn’t distinguish them, but psychographic profiles would.
By incorporating psychographic insights, companies gain a deeper understanding of what drives customer decisions. As one Harvard Business Review author noted, demographics tell us “who” the customer is, but psychographics reveal “why people buy,” often providing insight that complements basic demographic info. Psychological factors like personal values, political attitudes, risk aversion, or lifestyle preferences can heavily influence purchasing behavior. Modern segmentation that includes these facets leads to more resonant marketing: content and offers that align with customers’ inner motivations and identities.
Setbacks of Relying Solely on AI-Driven Segmentation
Let’s start with the fact that AI models (especially large language models and other pattern-based systems) can oversimplify human behavior. They excel at finding patterns in big data, but those patterns aren’t always meaningful or current. For instance, an AI might decide “Segment A” loves product features X and Y because of correlations in purchase data – yet it may ignore that within Segment A are different cultural backgrounds that view feature X very differently. The machine sees a data cluster; a human marketer might recognize it as an artificial grouping of distinct subcultures or emotional triggers.
Another concern is bias and loss of insight. AI models learn from historical data, and if that data is biased or incomplete, the segments will be too. Indeed, marketing researchers warn about “biased AI” and emphasize that some knowledge is tacit (intuitive know-how) and not easily transferred to AI.
Large models lack true human context: they don’t intuitively understand humor, irony, or emerging social trends until those show up in the data (which might be too late). This can lead to misinterpreting customer sentiment or missing emergent behaviors. Moreover, AI-driven segmentation can become a black box; if your team can’t explain why the algorithm grouped customers a certain way, it’s hard to build creative strategies or trust the output. Blindly following an AI’s segments might mean overlooking important emotional and cultural patterns that aren’t obvious in raw data.
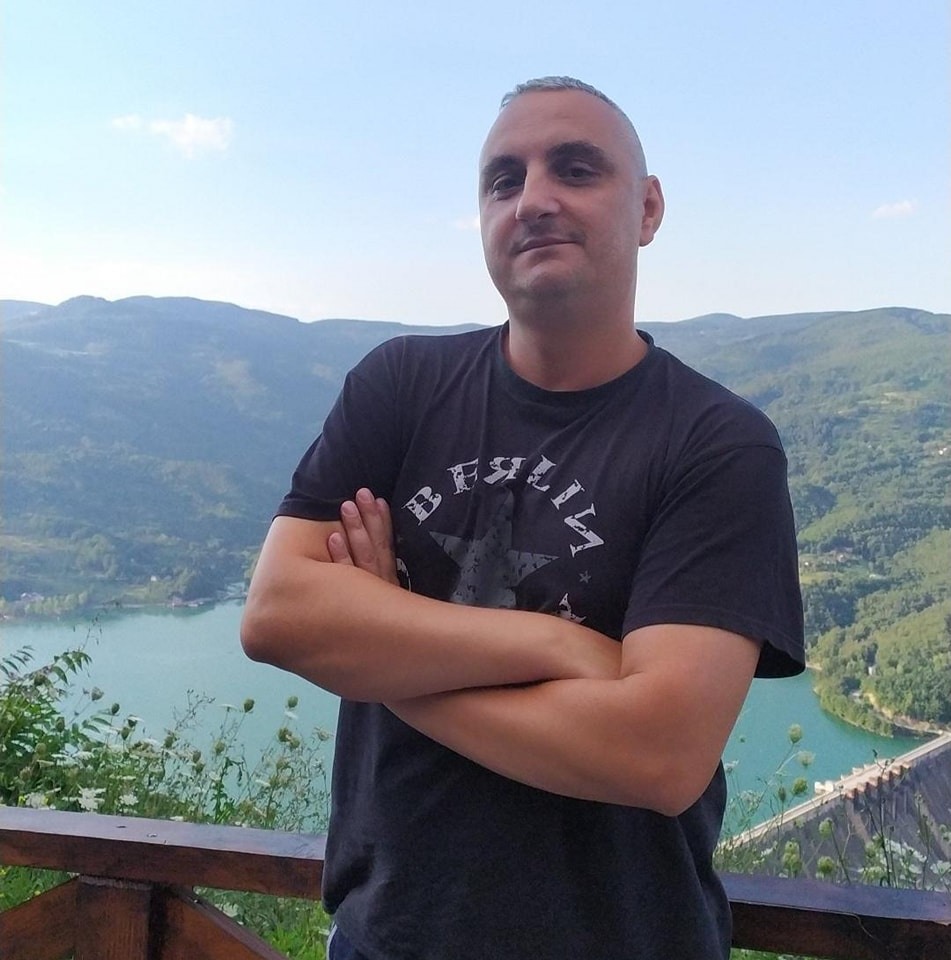
Andrej Fedek is the creator and the one-person owner of two blogs: InterCool Studio and CareersMomentum. As an experienced marketer, he is driven by turning leads into customers with White Hat SEO techniques. Besides being a boss, he is a real team player with a great sense of equality.